Keeping up with a fast-moving industry like AI is difficult. So, so that AI can do it for you, here's a handy summary of recent stories in the world of machine learning, along with notable research and experiments that we didn't cover on our own.
Last week, Midjourney, the AI-powered image (and soon video) creation startup, made a small, blink-and-you'll-miss-it change to its terms of service regarding the company's policy on intellectual property disputes. It essentially served to replace ridiculous language with provisions that were undoubtedly more legalistic and based on case law. But the change could also be seen as a sign of Midjourney's conviction that AI vendors like her will emerge victorious in courtroom battles with creators whose businesses include vendor training data.

Change to Midjourney's Terms of Service.
Generative AI models, like Midjourney's, are trained on huge numbers of examples — such as images and text — typically sourced from public websites and repositories on the web. Sellers confirm Fair use, the legal principle that allows copyrighted works to be used to create a secondary creation as long as it is transformative, protects them when it comes to model training. But not all innovators agree — especially in light of the growing number of studies showing that models can — and do — “rejuvenate” training data.
Some vendors have taken a proactive approach, signing licensing agreements with content creators and creating “opt-out” schemes for training datasets. Others promised that if customers got involved in a copyright lawsuit arising from their use of a vendor's GenAI tools, they wouldn't be on the hook for legal fees.
Midnight Flight is not one of those proactive ones.
Conversely, Midjourney has been somewhat brazen in its use of copyrighted works, at one point maintaining a list of thousands of artists—including illustrators and designers at major brands like Hasbro and Nintendo—whose works were, or would be, used. For training. Mid-flight models. One study shows convincing evidence that Midjourney used TV shows and movie franchises in its training data as well, from “Toy Story” to “Star Wars” to “Dune” to “Avengers.”
Now, there is a scenario in which court decisions ultimately go in Medjorney's favor. If the justice system decides to enforce fair use, nothing will stop the startup from continuing as it has been, mining and training on copyrighted data, old and new.
But this seems to be a risky bet.
Midjourney is flying high right now, having reportedly reached around $200 million in revenue without a dime of outside investment. But the cost of lawyers is expensive. If it was decided that fair use did not apply in Midjourney's case, it would kill the company overnight.
No reward without risk, eh?
Here are some other noteworthy AI stories from the past few days:
AI-powered advertising attracts the wrong kind of attention: Instagram creators criticized a director whose commercial reused other (more difficult and impressive) work without credit.
EU authorities are warning AI platforms ahead of the elections: they are asking the biggest companies in technology to explain their approach to preventing election hoaxes.
Google Deepmind wants your partner in cooperative games to be its own artificial intelligence: training the agent with many hours of 3D gameplay made it capable of performing simple tasks couched in natural language.
The Benchmarks Problem: Many AI vendors claim that their models have met or beaten the competition by some objective measure. But the metrics they use are often flawed.
AI2 Scores $200 Million: The AI2 incubator, spun out of the nonprofit Allen Institute for Artificial Intelligence, has a $200 million windfall in computing that startups going through its program can leverage to accelerate early development.
India requires government approval for AI, then backs away from it: The Indian government can't seem to decide what level of regulation is appropriate for the AI industry.
Anthropic launches new models: AI startup Anthropic has launched a new family of models, Claude 3, that it claims will compete with OpenAI's GPT-4. We put the flagship model (Claude 3 Opus) to the test, and found it impressive – but also lacking in areas like current events.
Political Deepfakes: A study by the Center for Countering Digital Hate (CCDH), a British non-profit, looks at the increasing volume of AI-generated misinformation — particularly election-related deepfakes — on X (formerly Twitter) over the past year.
OpenAI vs. Musk: OpenAI says it intends to reject all claims made by Company Impact on OpenAI's development and success.
Rufus review: Last month, Amazon announced that it would launch a new AI-powered chatbot, Rufus, within the Amazon Shopping app for Android and iOS. We got early access – and were quickly disappointed by how little Rufus can do (and does a good job).
More machine learning
Molecules! How do they work? AI models have helped our understanding and prediction of molecular dynamics, morphology, and other aspects of the nanoworld that would otherwise require expensive and complex methods to test. You still have to check, of course, but things like AlphaFold are changing the field quickly.
Microsoft has a new model, called ViSNet, that aims to predict so-called structure-activity relationships, the complex relationships between molecules and biological activity. It's still entirely experimental and certainly for researchers only, but it's always great to see difficult scientific problems being tackled with cutting-edge technology.
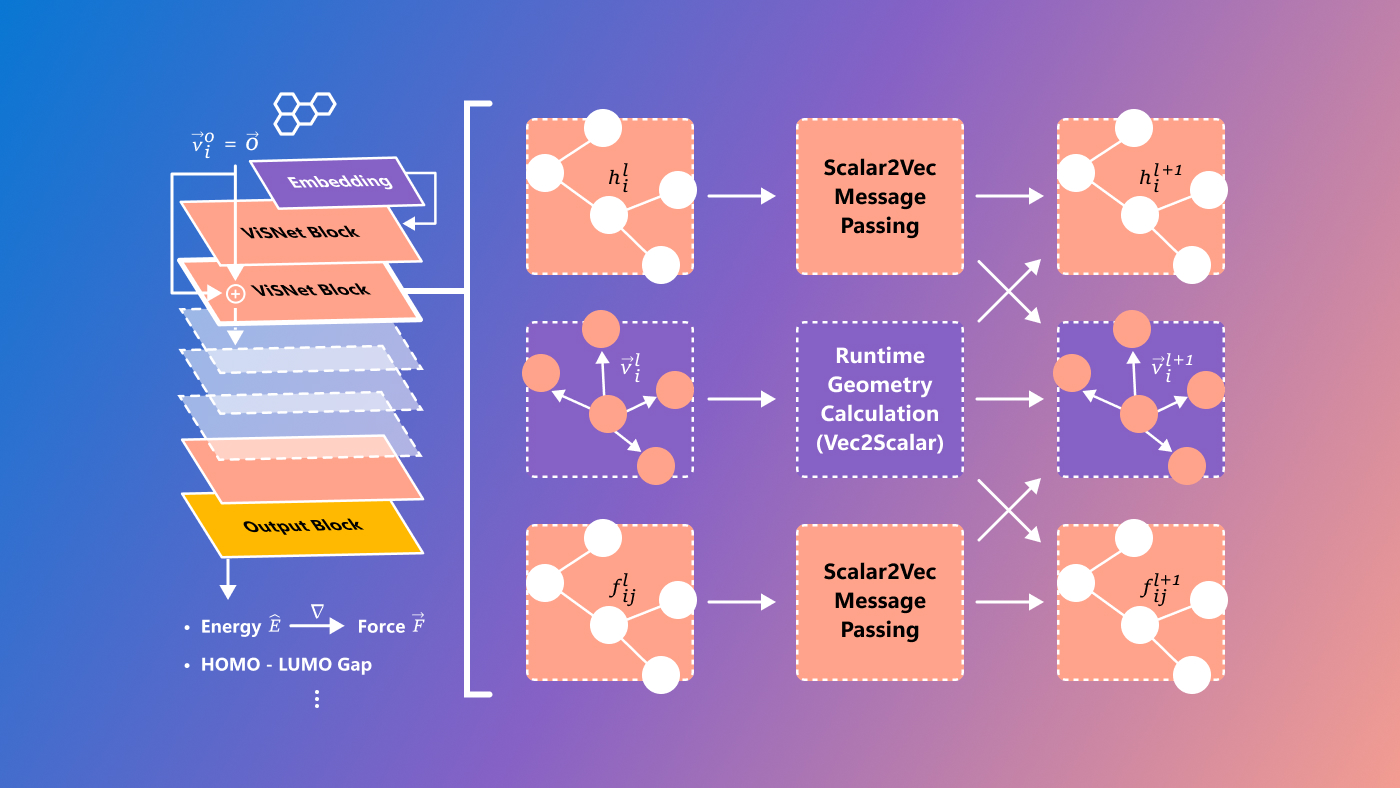
Image credits: Microsoft
Researchers at the University of Manchester are specifically looking at identifying and predicting coronavirus variants, less from a pure architecture like ViSNet and more through analysis of very large genetic datasets related to coronavirus evolution.
“The unprecedented amount of genetic data generated during the pandemic requires improvements in our methods to analyze it accurately,” said lead researcher Thomas House. His colleague Roberto Cahuanzi added: “Our analysis serves as a proof of concept, demonstrating the potential use of machine learning methods as an alert tool for early detection of key emerging variants.”
AI can design molecules as well, and a number of researchers have signed on to an initiative calling for safety and ethics in this field. Although David Becker (among the world's leading computational biophysicists) points out that “the potential benefits of protein design far outweigh the risks at this stage.” Well, as an AI protein designer, he would say so. But we must still be wary of regulation that misses the mark and hinders legitimate research while allowing bad actors freedom.
Atmospheric scientists at the University of Washington have made an interesting confirmation based on artificial intelligence analysis of satellite images over Turkmenistan over a period of 25 years. Essentially, the accepted understanding that the economic turmoil that followed the fall of the Soviet Union led to a decline in emissions may not be true – in fact, the opposite may have happened.
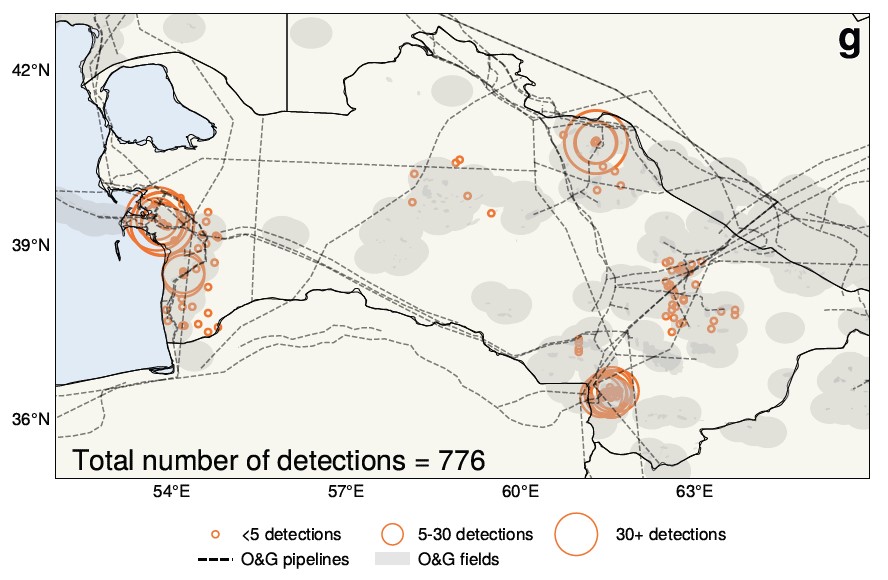
Artificial intelligence helped find and measure the methane leaks shown here.
“We found that the collapse of the Soviet Union seemed to lead, surprisingly, to an increase in methane emissions,” said Alex Turner, a professor at the University of Wisconsin. Large data sets and limited time to examine them made the topic a natural target for artificial intelligence, leading to this unexpected shift.
Large language models are largely trained on English source data, but this may further impact their ability to use other languages. EPFL researchers who looked at the “latent language” of LlaMa-2 found that the form seemed to revert to English internally even when translating between French and Chinese. However, the researchers point out that this is more than just a lazy translation process, and in fact the model has organized the entire underlying conceptual space around English concepts and representations. Does it matter? probably. We should diversify their data sets anyway.